Talking to Our Computers: The Rise of Natural Language Search
Cast your mind back to the late nineties, if you’re old enough, with the sound of your dial-up internet connecting as background music. You open AskJeeves and enter a question into the search bar: “Who are the spice girls?”
While AskJeeves may have faded in relevance in the face of the giant of all search engines, Google, this old-school search engine used principles of search that we’re now starting to see a swing back to: natural language, question-based search.
For years we’ve been searching Google using short keywords and phrases. But as the sheer amount of information on the world wide web increases exponentially, and Google (and other search engines) are constantly adapting to display the most relevant results to users’ search queries, we’re seeing a fundamental change in how search engines understand our queries.
And this change is in turn changing the way we search.
Image via Pixabay
The language of search engines
Think for a second about how you search. Chances are you’re using longer phrases, fuller sentences and more complex queries more frequently now.
Google is better and better equipped to deal with natural language, understanding that this is essential to deliver relevant results. Fernando Pereira, in charge of Google’s machine learning, said the “Most of our users interact with us through language. They ask queries, typed or spoken. And so for us to serve the user well, we have to make our systems understand what users want.”
The company has developed a number of tools to do this, part of a larger project in Artificial Intelligence. One of the latest releases is SyntaxNet, building on previous deep-learning frameworks. This tool learns the meaning of words and phrases in light of their context and how they are commonly used. Another tool is a pre-trained parser (a program that breaks text up into its various parts, such as nouns, verbs, subjects and objects). Hilariously named Parsey McParseface, this open-source parser is the most sophisticated of its kind yet in mastering the English language, by focusing on dependency grammar, or how words relate to each other in a given sentence. While it’s not 100% accurate, understandable given the incredible levels of ambiguity language allows for, it still achieves a remarkable 94% accuracy rate.
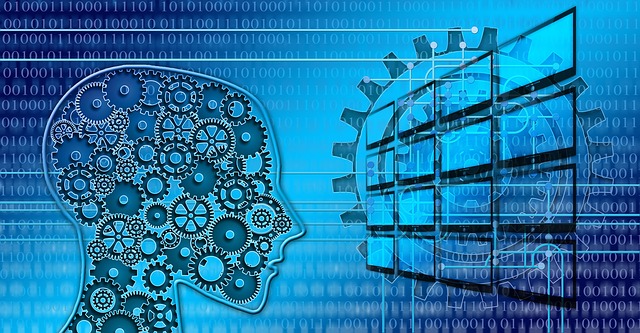
Image via Pixabay
This increased accuracy in understanding semantics can be seen in search results. Google introduced its Knowledge Graph in 2012, displaying information from users’ searches that quickly answers their queries without them having to click through to a specific page.
This Knowledge Graph has expanded to such an extent that it’s gone from understanding simple questions like “how old is Taylor Swift?” to “what are the ingredients for a screwdriver,” understanding that screwdriver in this context refers to the cocktail and not the tool. You can also throw in superlatives, dates and complex combinations such as:
“What was England’s population when the Queen was born?”
“Who was the U.S. president when the Angels won the World Series?”
“Who are the shortest NBA players?”
In other words, Google has made enormous strides in teaching its search bots to accurately understand what we mean when we enter our queries into the search bar.
Teaching machines additional languages
Interestingly, the language the search is in also influences the accuracy with which machines are able to understand. Some languages are easier to parse than others. Part of this is due to the syntax and how easy it is to break down a language into its different components. The more ambiguous a language, the harder it is to extract meaningful semantics.
Another factor is the amount of data available for machines to read and learn from. While English still reigns as the number one language on the web, this linguistic landscape is quickly changing, as website content in other languages proliferates. Currently leading the charge for most websites in their language (behind English), are Russian, German, Japanese, Spanish and French. Google is trying to keep up, having expanded their Knowledge Graph to encompass 15 languages so far.
Learning a second language? Check your level by taking a language test!
Search in the age of Siri
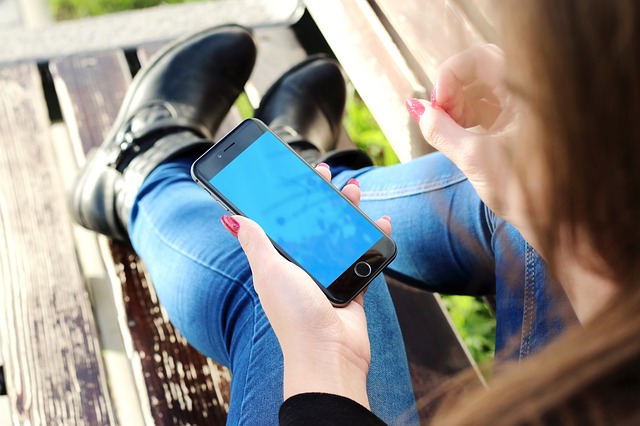
Image via Pixabay
Another factor driving the move to natural language search and teaching machines to understand the complexities of language is the rise of voice assistant technology like Apple’s Siri, Amazon’s Alexa, and Microsoft’s Cortana.
Voice technology has sped up the need for computers to understand conversational language. Think of the “Hey Siri” feature and her responses to personal questions. We’re encouraged to have conversations with our voice assistants, which has driven quick advancements in this field. This technology has its own added difficulties, with voice and accent variations that need to be deciphered on top of understanding sentence meaning. Anyone who has attempted voice commands has inevitably experienced frustrating and amusing misunderstandings with their voice assistant.
While huge advancements have been made in teaching computers to understand syntax, understanding semantics is still a challenge. Language, after all, is an enormously complex beast that is more than the sum of its parts.
Think of it as learning a second language. We’ve managed to teach computers grammar and vocabulary, but learning the subtleties, how native speakers use words in different contexts and the social and cultural aspects of language takes time. Will machines ever develop true fluency in human language? The jury is out.